Kolosov Ivan
Contact
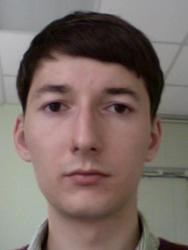
- Position:
- CMC MSU - Faculty of Computational Mathematics and Cybernetics of Lomonosov Moscow State University, Moscow
- Address
- Russia
Miscellaneous Information
- Miscellaneous Information
-
Abstract Reference: 30805
Identifier: P1.36
Presentation: Poster presentation
Key Theme: 1 Reduction and Analysis Algorithms for Large Databases and Vice-versa
Architecture of processing and analysis system for Big astronomical DataAuthors:
Gerasimov Sergei, Meshcheryakov Alexander, Kolosov Ivan, Glotov EvgenyNew discoveries in modern astrophysics are directly dependent on the quality of the algorithms for processing of a huge amount of astronomical images and event lists, produced by survey telescopes, and accurate methods for the their subsequent analysis. Currently, the volume of data collected through the digital sky surveys is experiencing the explosive growth. Per night the modern optical survey telescope can do up to a several thousands pictures of the night sky, providing the scientific data stream over 1TB / day. The rate of obtaining astronomical data will continue to grow exponentially with the next generation of survey telescopes coming into operation, such as LSST, WFIRST, EUCLID, eROSITA. Along with new sky surveys projects, the current amount of astronomical images stored in public astronomical archives already contains several petabytes of (mostly) imaging data. Our project is dedicated to creation the astronomical data reduction and analysis system based on (i) modern achievements in Big Data technologies (Hadoop and Spark ecosystem) and (ii) high-precision methods of machine learning analysis of big collections of data. The aim of our system development is to give to a single astronomer, or a small group of researchers, a powerful tool (readily available in the cloud), which enables them the consolidation of large volumes of multiwavelength imaging data and their unified processing into catalogs of sky objects, and a (ii) subsequent application of supervised and unsupervised machine learning algorithms for effective analysis.
Website of out group: www.astromining.org/publications