Barrientos Alejandro
Contact
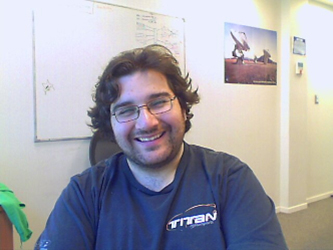
- Position:
- UTFSM - Universidad Tecnica Federico Santa Maria, Santiago
- Address
- Chile
Miscellaneous Information
- Miscellaneous Information
-
Abstract Reference: 30241
Identifier: P2.2
Presentation: Poster presentation
Key Theme: 2 Management of Scientific and Data Analysis Projects
Machine Learning approaches for detection and classification of astrochemical spectral lines (lightning talk)Authors:
Barrientos Alejandro Javier Solar MauricioAstronomical spectroscopy is a field that has been growing for a number of years, analyzing the features of molecular spectral lines from astronomical data cubes provides insight to the composition and dynamics of our universe. With the arrival of state-of-the-art high spectral resolution radiotelescopes like ALMA, the size of the data cubes will be constantly growing in time. This is why we believe that some automatic analysis methods will be helpful assisting the astrochemists work. We would like, to generate a method to analyze astronomical data cubes, detect their regions of interest, by using a non supervised clustering algorithm, and then, generate a spectrum for each region of interest, and classify the molecular species found in the spectra, by using a supervised training algorithm. The training for the learner is done using synthetic spectra, and the validation is done using radio astronomical data cubes from ALMA observational data. Initial experiments contemplated a naive physical model that was considered to start the problem and two popular Machine Learning methods were tested for the task of classifying molecular spectra, Support Vector Machines and Neural Networks Results for classification accuracy for the testing data ranged between 86.2 and 99.9 percent. We conclude that the problem is more complex than classifying laboratory spectra, also, the scarcity of real-world examples makes synthetic data useful to test the models. Our first approach provides the foundations for more complex models, and, as we keep adding physical parameters into the model, the need for a more potent approach, such as the Machine Learning approach, becomes clearer.