Park Jongyeob
Contact
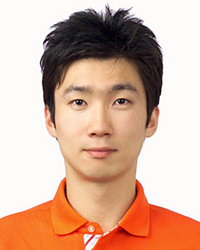
- Position:
- Korea Astronomy and Space Science Institute, Daejeon
- Address
- South Korea
Miscellaneous Information
- Miscellaneous Information
-
Abstract Reference: 31070
Identifier: P1.23
Presentation: Poster presentation
Key Theme: 1 Reduction and Analysis Algorithms for Large Databases and Vice-versaDevelopment of a forecast model of solar wind speed using the convolution neural network
Authors:
Park Jongyeob, Moon Yong-Jae, Cho Il-Hyun, Choi Seonghwan, Baek Ji-Hye, Cho Kyung-SukThe Solar Dynamic Observatory (SDO) produces the huge size of scientific imaging data of the Sun from AIA and HMI. The Korea Data Center for SDO (KDC-SDO) has stored the full coverage data of the SDO which are identically same in Joint Science Operations Center (JSOC). We develop a preliminary forecast model of solar wind speed (Vsw) using the convolution neural network (CNN) in the Tensorflow of Google. For this, corona holes are identified as dark area whose intensity are lower than median value using AIA 193Å images. The input data for CNN are binary images with -10 and 10 degrees in longitude: 1 for corona holes and 0 for the other areas. Three day data with 1 hour time resolution are used for the solar wind speed forecast with one day advance. The forecasting events are defined as positive samples which are Vsw>500km/s for the whole day. The number of positive samples are 204 from 2010 to 2016. The CNN is configured to 1 convolution layer, 1 pooling layer, and 1 fully-connected layer that has 4 nodes at last. The forecast model can classify the events to happen or not. With K-folds cross-validation in a training process, the average of accuracy for the model is 0.49 and its standard deviation is 0.12. Finally, the model is evaluated within a month and its accuracy is 0.54. We will use SOHO EIT images for more training samples, and SOHO LASCO images to consider coronal mass ejections. In addition, we can extend this study to forecast other solar and geomagnetic parameters.